The Role of AI in Modern Trading
Discover how AI is transforming modern trading with advanced algorithms, market prediction models, and ethical considerations shaping the future of financial markets.
AI
Share
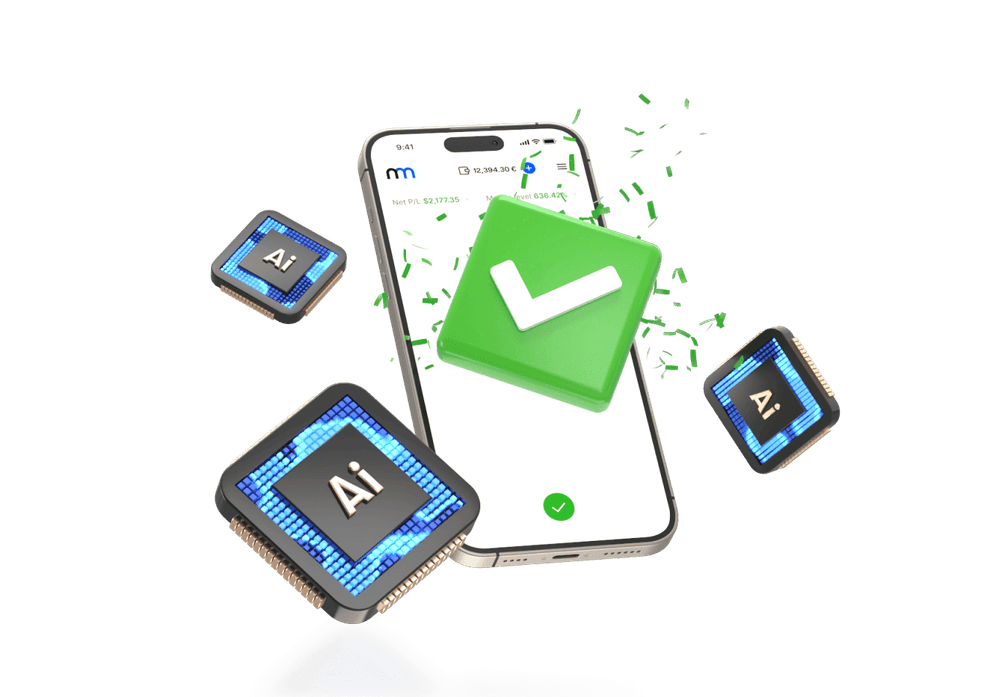
Artificial Intelligence (AI) 🤖has rapidly transformed the landscape of financial markets, with its impact on trading becoming increasingly profound.
According to a 2023 report by Grand View Research, the global AI in fintech market size was valued at $9.45 billion in 2022 and is expected to grow at a compound annual growth rate (CAGR) of 16.5% from 2023 to 2030.
This staggering growth reflects the pivotal role AI now plays in modern trading practices. This article explores the evolution of AI in trading, its current applications, the technologies driving its adoption, and the challenges and ethical considerations that arise from its use.
Introduction to AI in trading 📈
The integration of AI in trading can be traced back to the 1980s when early forms of algorithmic trading began to emerge. In 1983, the New York Stock Exchange introduced the Designated Order Turnaround (DOT) system, which allowed for the electronic transmission of orders, paving the way for more sophisticated trading algorithms. However, it wasn't until the late 1990s and early 2000s that AI started to make significant inroads into trading practices.
A major milestone came in 2005 when the New York Stock Exchange merged with Archipelago, an electronic trading platform, signaling a shift towards more automated trading systems. By 2010, high-frequency trading (HFT) firms, heavily reliant on AI algorithms, accounted for more than 60% of all U.S. equity trading volume. The flash crash of May 6, 2010, where the Dow Jones Industrial Average plunged about 9% in mere minutes before rapidly recovering, highlighted both the power and potential risks of AI-driven trading systems.
Since then, advancements in machine learning, natural language processing, and big data analytics have further refined AI's capabilities in trading, leading to more sophisticated predictive models and risk assessment tools.
AI-powered trading strategies and algorithms 🤖
The advent of AI 🖥️has revolutionized trading strategies, enabling more sophisticated and responsive approaches to market dynamics. These AI-driven algorithms can process vast amounts of data in real-time, identifying patterns and trends that human traders might miss. For instance, JPMorgan's LOXM algorithm can execute trades with maximum speed and minimum market impact, showcasing the efficiency of AI in high-frequency trading.
Some key AI-powered trading strategies include:
- Sentiment analysis of social media and news
- Pattern recognition in price movements
- Predictive modeling for market forecasting
- Automated portfolio rebalancing
- Arbitrage detection across multiple markets
- Risk management and fraud detection
These strategies leverage machine learning algorithms that continuously adapt to changing market conditions. A notable example is Renaissance Technologies' Medallion Fund, which has used AI-driven quantitative models to achieve annual returns of 66% before fees from 1988 to 2018. The success of such AI-powered funds has led to their increased adoption, with a 2022 Refinitiv report indicating that 78% of trading firms are now using or planning to use AI in their trading strategies.
Machine learning models for market prediction and risk assessment 📊
Machine learning models have become indispensable tools for market prediction and risk assessment in modern trading. These models, ranging from simple linear regression to complex neural networks, analyze historical data to forecast future market trends and potential risks. For example, gradient boosting algorithms, such as XGBoost, are widely used for predicting stock prices due to their ability to handle non-linear relationships in financial data. Similarly, Long Short-Term Memory (LSTM) networks, a type of recurrent neural network, have shown remarkable accuracy in forecasting time series data like stock market movements.
In risk assessment, machine learning models are employed to evaluate credit risk, detect fraudulent activities, and assess market volatility. Banks and financial institutions use these models to automate loan approval processes and determine credit limits. For instance, JPMorgan Chase developed a machine learning model called COiN (Contract Intelligence) that can review commercial loan agreements in seconds, a task that previously took 360,000 hours of work by lawyers and loan officers annually. In the realm of market volatility, reinforcement learning algorithms are being utilized to optimize trading strategies in response to changing market conditions, allowing traders to adapt their positions dynamically to minimize risk and maximize returns.
Natural language processing in financial news analysis 📰
Natural Language Processing (NLP) has emerged as a crucial tool in financial news analysis, enabling traders to rapidly process and interpret vast amounts of textual data. NLP algorithms can analyze news articles, social media posts, and corporate filings to gauge market sentiment and predict potential market movements. For example, hedge funds like Two Sigma and Renaissance Technologies employ sophisticated NLP models to scan thousands of news sources in real-time, extracting relevant information that might impact their trading decisions.
These NLP systems can detect subtle nuances in language that might indicate positive or negative sentiment towards a company or industry. They can also identify emerging trends or potential risks before they become widely known. For instance, some NLP models are capable of analyzing earnings call transcripts to predict a company's future performance based on the language used by executives. This ability to quickly process and act on textual information gives AI-driven trading systems a significant edge in today's fast-paced markets.
Challenges and ethical considerations in AI-driven trading ⚖️
While AI has revolutionized trading, it also presents significant challenges and ethical concerns. Some key issues include:
- Algorithmic bias and fairness
- Lack of transparency in AI decision-making
- Potential for market manipulation
- Cybersecurity risks
- Job displacement in the financial sector
- Regulatory compliance and accountability
One of the primary challenges is ensuring the fairness and unbiased nature of AI algorithms. Machine learning models can inadvertently perpetuate or amplify existing biases present in historical data, potentially leading to discriminatory trading practices. Additionally, the "black box" nature of some advanced AI models makes it difficult for regulators and investors to understand how trading decisions are made, raising concerns about transparency and accountability.
Another significant challenge lies in the realm of data privacy and security. AI trading systems rely on vast amounts of data, including sensitive financial information. Ensuring the protection of this data from cyber threats and unauthorized access is crucial. Moreover, the use of alternative data sources, such as satellite imagery or social media sentiment, raises questions about privacy boundaries and the ethical implications of using such information for financial gain.
Regulatory bodies are grappling with how to effectively oversee AI-driven trading. The complexity and opacity of some AI models make it difficult for regulators to understand and audit these systems. There's also the ongoing debate about the level of human oversight required in AI trading systems and where the line of accountability should be drawn when AI-driven decisions lead to significant market events.
Summary 📌
- AI has revolutionized trading strategies, enabling more sophisticated market analysis and faster execution of trades.
- Machine learning models are being extensively used for market prediction and risk assessment, improving accuracy and efficiency in trading decisions.
- Natural Language Processing has become a crucial tool in analyzing financial news and sentiment, providing traders with valuable insights from textual data.
- The adoption of AI in trading presents significant challenges, including potential market volatility, data privacy concerns, and regulatory complexities.
- Ethical considerations surrounding AI-driven trading, such as algorithmic bias and market fairness, necessitate ongoing dialogue and development of responsible AI practices in the financial industry.
Share
Similar articles